or, if open science were Wordle we might usually get the answer on the first line.

This is a blog that compares science (the open kind) to cheating at Wordle. But not in a bad way. This is a blog, so I’ll run the narrative first. I have included links to further readings from The Open Scientist Handbook. [OSH] You can find all the other literature references added at the end.

ASAPbio recently (October, 2022) announced a competition to share negative results as preprints. <https://asapbio.org/competition2022>. Sharing negative results is integral for open science to achieve its unreasonably effective potential. The sharing of all research products is one of open science’s main goals.
We can imagine, in an alternative present, an academic publishing endeavor that has long made space for null-results. Ideally, these results would be available in the same journals that publish positive results, and in the same proportion as these are each generated through rigorous scientific methods. Publication in this manner might fairly accurately reflect the sum of knowledge generated by research (if it included data, software, etc.).
Now, let’s look at the academic publishing regime we have today, where null-results are conspicuously absent, and the published corpus reveals a tiny fraction of the work of scientists across the globe. Sources on the topic of “publication bias” outline how this damages the entire academy. A further assortment of bad practices — of bad science — can also be uncovered through methodological and content reviews of published research. This is where Retraction Watch comes into play.
We can find at least two streams of perverse incentives in the current publication situation. The first is an outcome of the arbitrary scarcity of publication opportunities. This warps the whole research landscape and rewards narrowly selected research results, instead of valorizing methodological rigor. Even the available published work rarely includes enough information to allow replicating the research.
The second stream of bad science is the central role the current publication regime plays in career advancement and future funding. By using metrics that are hooked into “journal impact factors,” and other forms of pseudo prestige (e.g., the h-index), universities and funders get to pretend they can evaluate the merits of a researcher’s work without needing to spend the time and effort to make an actual qualitative review.
Apart from the weaknesses inherent in this metric, simply as a metric, when this metric becomes a goal for researchers, Goodhart’s Law predicts that this will be gamed until its original value is erased or even reversed. The unnecessary scarcity of publication opportunities creates an ersatz elite of “published” academics, and a much larger cohort of undervalued, marginalized researchers. [Fierce equality] (Perhaps another blog is needed to show how academy publication is like TikTok.)
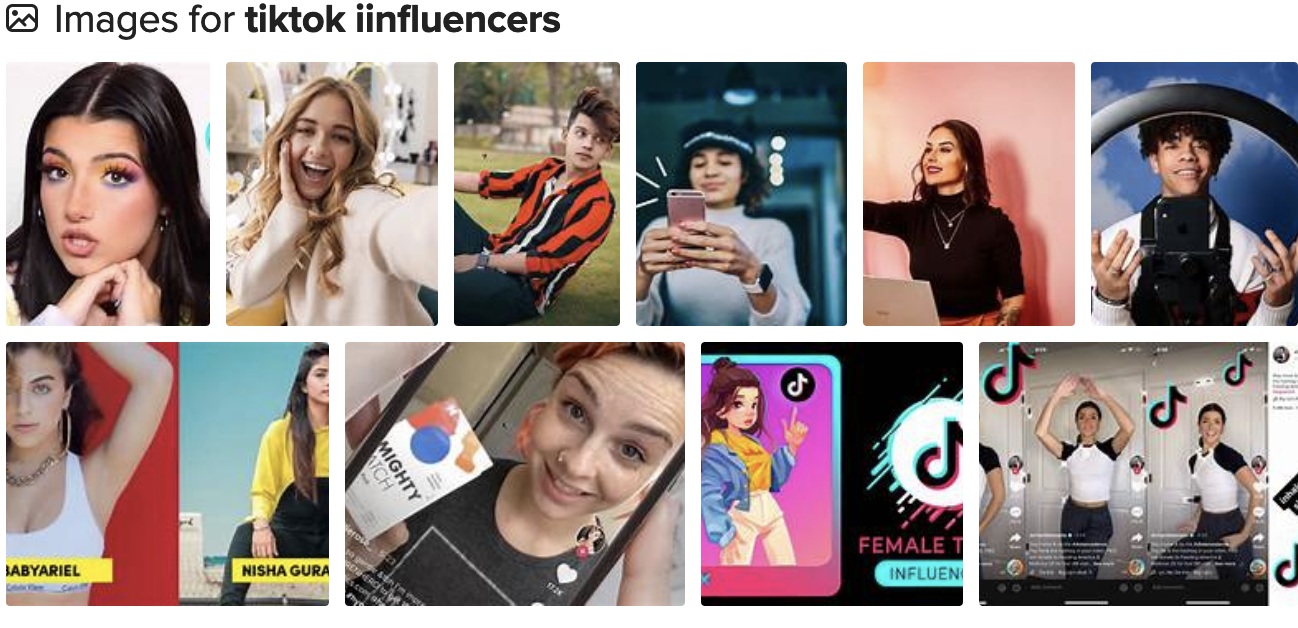
At the same time, the race to get published crowds out the open sharing of research results. The need to be first also prevents collaborative networked interactions with other teams that could greatly accelerate new knowledge discovery. When the great majority of the actual work of science has no place to be shared, the global work of science is fundamentally diminished.
The current availability of preprint servers for a number of disciplines (and also new AI search engines to facilitate discovery) means we are at the front edge of the capability to demonstrate how widespread, open sharing can decenter the current logic of scarcity, in favor of a new, extraordinary abundance.
Back to Wordle
I am going to use Wordle as an analogy that can show the unreasonable effectiveness of open sharing.
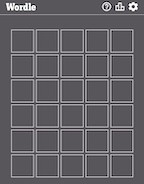
For those of you who don’t Wordle, when you start a Wordle puzzle, you have six chances to uncover the correct five-letter word. Each layer provides information to help you out with the next layer.
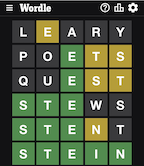
You build a solution space for the correct answer by knowing which letters are not used and which are used in the wrong place, or in the correct place.
That’s right. You get to use your own mistakes to learn and improve. In every new layer, the puzzle gets easier. There is also guesswork, and so a bit of luck involved. It’s an elegant design for a short puzzle. You can do one a day.
If Wordle had eight layers, most players would never lose. If Wordle only had two layers, most players would rarely win. Wordle works because its difficulty level is a sufficient challenge to most who play it.
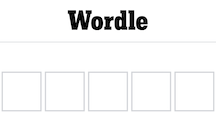
But what if your job future depended on you filling in the correct word on the first level? What if you are given this puzzle and told you must guess correctly or find a new position?
You might be tempted to look online and find the answer. Everyone solves the same puzzle each day. Cheat to win? Why not? You can do “bad Wordle.” But then, someone would add a metric based on time, and only the first person who solves the puzzle gets job security. And on and on. The zero-sum-game solution.
However, unlike Wordle, the science puzzle in front of you has never been solved. That’s the whole point. You choose a significant unknown, because that is why you do science. You need to solve something new.
Currently, within the arbitrary scarcity of the publication regime, you need to solve this unknown now, because others are out there looking to solve the same, or related problems. Only the first solution will get published. It’s your lab team against all the others out there. (Of course this is an unnecessary competition, and a hallmark of failed science, but that’s another blog, comparing science with Survivor.) [Playing the game]
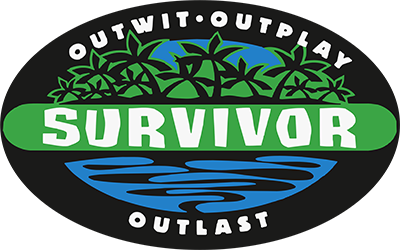
When you propose a research experiment, you only get one line: you have one chance to discover a result that explains something new. Nature (actual nature, not the journal) doesn’t give you a lot of hints, and the NSF has (finally) funded this one project for your team.
You are a scientist. You have subscribed to the hardest puzzle anywhere. Your job is to provide the answer to this puzzle. You have lined up all the resources you believe are sufficient. You have a proven methodology and a plan. Your team does the work. You have your results. Now you must publish your work.

Let’s say that getting an article into the academic journal of your choice (the one with a “high impact factor,” or whatever) today requires this:

After finishing the project your actual research result, the information you found, may look like this:

Each bit of that finding is as valuable to science as any other finding. It just has no public home to go to. Today you have one sort-of-good — but actually unfortunate — choice, and other “choices” that are not good at all, and totally unfortunate for science.
The “goodish” choice is to keep the data safe, maybe host it up on a repository, and do the write-up for the granting agency. Use the lesson learned for the next research project. Your lab will add this research to its shared knowledge and move ahead. This is the “file-drawer” outcome.
You will wonder how this outcome will affect your ability to get future funding, and realize the lack of a publication might impact your next performance review. You might feel like all the work you accomplished was wasted. Yes, you found out something new, something important on its own terms, only this result was “negative.” It does not spell out a “significant finding” you can use to leverage your career.
Your research revealed a new piece of the larger truth. In terms of the knowledge space of your field, this new information occupies corner of the space of “already-accomplished-research.” It is not any less significant as a finding than any other research. It is another step in the long journey that is science. [Science and the infinite game] However, today, this work matters far less than it should to the academy. And less than it might for your job.
At this point, all of the perverse incentives of current science are now clearly in play if you let them infect what you do next. [Toxic incentives] Perhaps, in some desperation, you go back to the data and revise your hypothesis to match the “findings.”
Maybe you set out to prove that “people who eat their largest meal in the morning gain less weight,” but now your research proves — with great statistical precision — that “people do not eat while they are asleep.”
You shop this “finding” around to journals and one of them publishes it. It is no longer research you are proud of, but your list of publications is larger, and your funder might not notice.

Maybe you cannot find a different hypothesis, so you go back to the data and “regularize” this until some significant pattern pops up. You announce this as a “finding” and shop it to journals. Your lab team will need to be in on this move. They are implicated in your deceit. You figure that nobody will get funded to replicate your work, so this finding will be accepted as legitimate.

Congratulations, your published work will mislead everyone who cites it. You have wounded the body of knowledge you and your colleagues share. Your career now means more to you than the integrity of your work.
Open science can fix this. It must, and it will.
Here is where open science can help. Let us imagine that the academy has promoted publishing null-results on preprint and eprint servers for a decade. With no need for the “file-drawer” option, the number of null-result findings available online is now much larger than the number of recent significant findings. (Note: I use the term “eprint” the signify new publishing efforts that publish all submissions and then do open peer review to add value to these.)
Because nobody needs to contort their research to get published, the actual research statistics being used are much more rigorous, and the data more reusable and available. Let’s add here that a null-result pre/eprint that gets cited is treated the same as any other publication, in terms of career-building metrics. That’s another goal of open science: new institutional cultural practices and norms.
Back to square one… you still need to do the research

You have just received notice that your funding has been approved. You are still faced with a complex phenomenon to explain, just as before.
Of course, you have already done a complete literature search through all the appropriate journals to see if there are positive findings that would improve the questions you have, and the final hypothesis you will be using. Your research did not end with the already-published positive findings. Before you even wrote you proposal, you expanded your research (using powerful AI-enhanced search routines, and advanced keyword techniques) to include negative-results in related experiments. These are mostly posted on preprint servers.
This is what you discovered: A colleague of yours in Germany did a research project closely related to your work, and this was their team’s result:

Another colleague in China also put their negative result up on a preprint server:

A post-graduate researcher in California submitted their research findings to a preprint server:

Your team sifts through these findings and their open data. You use protocols developed to match other findings with the phenomenon you are investigating.
You are encouraged when you realize that instead of just this:

The shared resource of null-results has filled in many of the unknowns internal to the unknown you are tackling. You now know so much more about the object/process under study:

You have a better handle on the problem you face. Your team can focus its methods on only those parts that are missing. The rest of the puzzle offers new clues to its solution. You really only need one line to figure this out.

You now have a much better starting point, a major advantage, from which to discover something that completes a bit of the landscape of current science knowledge.

Your agency’s program manager is jazzed. Your university puts out a press release. You are networking with new collaborators across the globe, planning the next project together. Of course, you cite the research of all of your sources in your publication. Their shared research products made your findings possible. [Demand Sharing] You add your data to theirs on an open repository. And you pop the resulting publication onto an open, online server.
Science is hard enough. Let’s work in our universities, agencies, and societies to promote the added, unreasonably effective, benefits of open sharing and collaboration.
Certainly the open data you discover from the null-research results cannot be expected to be quite so providential for your work. But these shared resources will offer an abundance of new information and helpful guidance for your own efforts. You are not alone. You don’t have to race. There is no race to win. Your lab has posted seventeen prior research results with data — all of them negative results — up on the web. Your grad students field requests for these data and collect citations for this work. They are making connections across the planet that should enhance their future careers. Curiously, without the race, science moves a lot faster.
A hundred-thousand science research teams working apart, each one of them looking to “win science” by keeping their work secret, would fail constantly against a hundred teams working in concert. [Open Collaboration Networks] The latter gain insights and save time by sharing all of their work toward a common goal of collective understanding.
The unreasonable effectiveness of shared null results is just one example of how embracing abundance instead of scarcity accelerates science knowledge discovery.
CODA: Free riders on the sharing-null-results bus
There is a “what’s wrong with this picture” perspective we can clear up, even if we don’t have an optimal solution space (that space will need to be emergent). Any move from a zero-sum game (e.g., science today) to a non-zero-sum game, allows a few zero-sum game players — those who don’t mind violating cultural norms for their own advantage — to add the shared non-zero-sum assets to their own work, without attribution, and potentially compete more efficiently than before. This is your basic “free-rider” problem. Every commons faces this problem.
Looking at this another way, the free-rider problem becomes a free-rider opportunity within the academy, as long as the cultural norms for sharing are present. [Share like a scientist] Every scientist is a “free-rider” on the discoveries they use in their own research. The real free-rider problem happens when open resources are acquired freely and aggregated by corporations, which want to sell these back to the academy as proprietary property, with some marginal value-added service.
Free-riding is a problem that culture change can help resolve. Yes, there will be those who grab these assets and use them without credit, or massage these and market them. The general strategy for jerks, those who take advantage of a positive cultural change that valorizes sharing, is to marginalize them wherever possible. Academic institutions can cultivate social outrage against those who plagiarize others’ work, including null-results. Agencies can fund open repositories, and require their use. Open means really open. Closed, as John Wilbanks reminded us, means broken.
Additional readings and quotes from them
Bibliographic citations here
On publishing not capturing what science knows, and what reuse requires:
“In present research practice, openness occurs almost entirely through a single mechanism — the journal article. Buckheit and Donoho (1995) suggested that ‘a scientific publication is not the scholarship itself, it is merely advertising of the scholarship’ to emphasize how much of the actual research is opaque to readers. For the objective of knowledge accumulation, the benefits of openness are substantial…
Three areas of scientific practice — data, methods and tools, and workflow — are largely closed in present scientific practices. Increasing openness in each of them would substantially improve scientific progress.”
Nosek, Spies, and Motyl (2012); Buckheit and Donoho (1995)
On publication bias:
“Publication bias is a common theme in the history of science, and it still remains an issue. This is encapsulated in a piece of commentary published in Nature: ‘…negative findings are still a low priority for publication, so we need to find ways to make publishing them more attractive’ (O’Hara, 2011). Negative findings can have positive outcomes, and positive results do not equate to productive science. A reader commented online in response to the points raised by O’Hara: ‘Imagine a meticulously edited, online-only journal publishing negative results of the highest quality with controversial or paradigm-shifting impact. Nature Negatives’ (O’Hara, 2011). Negative results are considered to be taboo, but they can still have extensive implications that are worthy of publication and, as such, real clinical relevance that can be translated to other related research fields.”
Matosin, et al (2014); O’Hara (2011)
On impact factors and the h-index:
“Funders must also play a leading role in changing academic culture with respect to how the game is played. First and foremost, funders have a clear role in setting professional and ethical standards. For example, they can outline the appropriate standards in the treatment of colleagues and students with respect to such difficult questions as what warrants authorship and how to determine its ordering. Granting agencies should clearly emphasize the importance of quality and send a clear message that indices should not be used, as expressed by DORA, which many agencies have endorsed. Of particular importance is for funders not to monetize research outputs based on metrics, such as the h-index or journal impact factor.”
Chapman, et al (2019)
On Goodhart’s Law:
“The goal of measuring scientific productivity has given rise to quantitative performance metrics, including publication count, citations, combined citation-publication counts (e.g., h-index), journal impact factors (JIF), total research dollars, and total patents. These quantitative metrics now dominate decision-making in faculty hiring, promotion and tenure, awards, and funding.… Because these measures are subject to manipulation, they are doomed to become misleading and even counterproductive, according to Goodhart’s Law, which states that ‘’when a measure becomes a target, it ceases to be a good measure’”.
Edwards and Roy (2016)
On the file-drawer problem:
“For any given research area, one cannot tell how many studies have been conducted but never reported. The extreme view of the ‘file drawer problem’ is that journals are filled with the 5% of the studies that show Type I errors, while the file drawers are filled with the 95% of the studies that show non-significant results. Quantitative procedures for computing the tolerance for filed and future null results are reported and illustrated, and the implications are discussed.”
Rosenthal (1979)
On Science and the Infinite Game:
“The paradox of infinite play is that the players desire to continue the play in others. The paradox is precisely that they play only when others go on with the game. Infinite players play best when they become least necessary to the continuation of play. It is for this reason they play as mortals. The joyfulness of infinite play, its laughter, lies in learning to start something we cannot finish”
Carse (1987).
On the free-rider problem:
“But here’s the thing. In addition to the free rider problem, which we should solve as best we can, there’s a free rider opportunity. And while we whine about the problem, the opportunity has always been far larger and its value grows with every passing day.
The American economist Robert Solow demonstrated in the 1950s that nearly all of the productivity growth in history — particularly our rise from subsistence to affluence since the industrial revolution — was a result not of increasing capital investment, but of people finding better ways of working and playing, and then being copied.”
Gruen: We’re All Free Riders. Get over It!: Public goods of the twenty-first century